This New AI Architecture Could Transform the Government
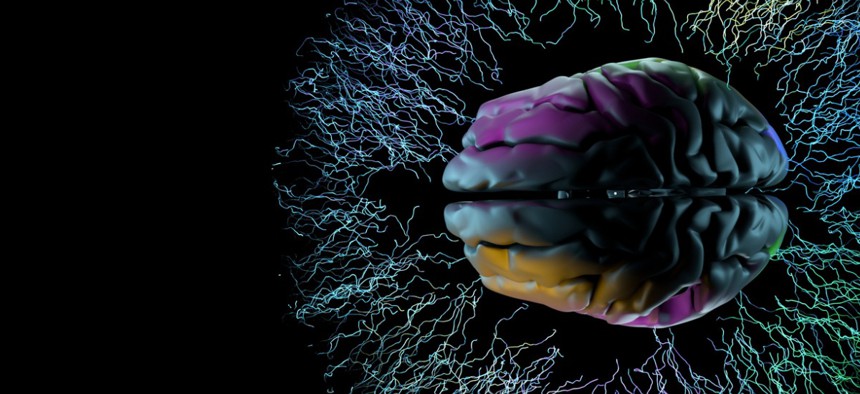
Just_Super/iStock.com
Neuromorphic computing has a long way to go but could help the government respond to a multitude of situations with greater ease, speed and sophistication.
Last year, the Department of Energy announced $2 million in funding for research on neuromorphic computing, which aims to mimic the structure and functionality of the human brain. This new approach could provide a more efficient and malleable way to process data in ways not yet seen in the computing world. For government agencies, that could open new use cases, lower power consumption, and potentially save both money and lives.
Agencies are already using traditional artificial intelligence to complete their missions and make decisions. But early generations of AI were built as binary systems. A system that emulates human cognition allows AI to be used for more complex tasks and in more abstract situations. From a mission perspective, neuromorphic computing has the potential to breed improved accuracy and better predictive capabilities than a traditional deep learning network—and the industry continues to look at lowering the costs. With neuromorphic computing not only automation, but self-learning autonomy is possible within a very efficient power envelope.
Let’s dive a bit deeper into this next generation of AI, and how it could impact government operations.
A New Neuromorphic Architecture
The first generation of AI used rules to draw reasoned conclusions within a specific, narrowly-defined problem domain. Such AI is well-suited for monitoring. Even more sophisticated AI, like deep learning, processes information in sequence, in the same vein as a typical computer program. But neuromorphic computing allows for granular event-based asynchronous processing. Think of this as the marriage of neuroscience with computer science. Like the human brain, neuromorphic computing can evaluate different scenarios all at the same time. This means a far more comprehensive self-learning neural network, giving even more potential for parallel computation that is much greater than today’s GPUs.
Put another way, neuromorphic computing aims to use computer science to reframe classical logic-based AI into more flexible systems. As is the case with human cognition, these systems can contextualize interpretations and adapt to them autonomously. In turn, algorithms can deal with uncertainty and change almost as effectively as humans. Neuromorphic computing has the potential to require less power making it a particularly savvy technological investment in our current climate crisis.
This opens a world of high-functioning and self-learning AI, comfortable in a wide range of situations and extremely relevant to the government space. It also comes with challenges. Neuromorphic computing isn’t just a paradigm shift; it requires a near-complete restructuring of current technology: redesigned chips, servers, and typical supercomputer infrastructures. But what it provides could well be worth the effort.
Government Use Cases
Agencies are collecting, processing, and storing more data than ever thanks to the growing prominence of sensor technology. Next-generation AI must be able to address novel situations and abstraction to make the most of all this data. With neuromorphic computing, the neural network could take over a greater portion of analysis for logistics planning, resource planning and optimization, image classification and reconstruction, and problems that consider a wide range of variables in parallel.
With neuromorphic computing, researchers are evaluating how to fuse computer vision and touch so computers can better understand gestures. Neuromorphic architectures can also be programmed so that their dynamic behavior over time mathematically optimizes specific objectives. This could be applied to maximize the bandwidth of a wireless channel, for instance. And once humans define the constraints in an application or database of learning, neuromorphic systems can accelerate insights by exploring many different solutions in parallel at high speed, allowing government agencies to quickly search for a needle in a haystack or evaluate different mission scenarios.
Neuromorphic computing can also help government agencies collect data, particularly in dangerous places. Whether we are trying to land on Mars or explore the ocean’s depths, there are certain places that present a high risk to humans. With neuromorphic computing, AI could actually pilot vehicles on data-gathering missions, in turn potentially saving human lives. The Challenger disaster would have been less of a disaster if humans weren’t in it. But in order to have AI pilot such trips, it has to be able to learn and react, which requires a neuromorphic approach.
The Bottom Line
Neuromorphic computing seeks to emulate the complexities and capabilities of our most treasured computer found in nature. The human brain is roughly a million times more energy-efficient than the most advanced supercomputers in existence today. Neuromorphic computing could be particularly impactful in the government space, with agencies responsible for so many aspects of human life.
Neuromorphic computing requires an entire paradigm shift and new computing architecture. Put simply, it still has a long way to go. But looking forward, this next generation of AI has tremendous potential to help the government respond to a multitude of situations with greater ease, speed and sophistication.
The government has already made great strides from a technological perspective and is working hard to use all the data it’s collecting. Neuromorphic computing can make that task even simpler, as its algorithms can handle complexity just like a human.
Gretchen Stewart is chief data scientist of Intel Public Sector.